
Iceberg supports Apache Spark for both reads and writes, including Spark’s structured streaming. Initially released by Netflix, Iceberg was designed to tackle the performance, scalability and manageability challenges that arise when storing large Hive-Partitioned datasets on S3.
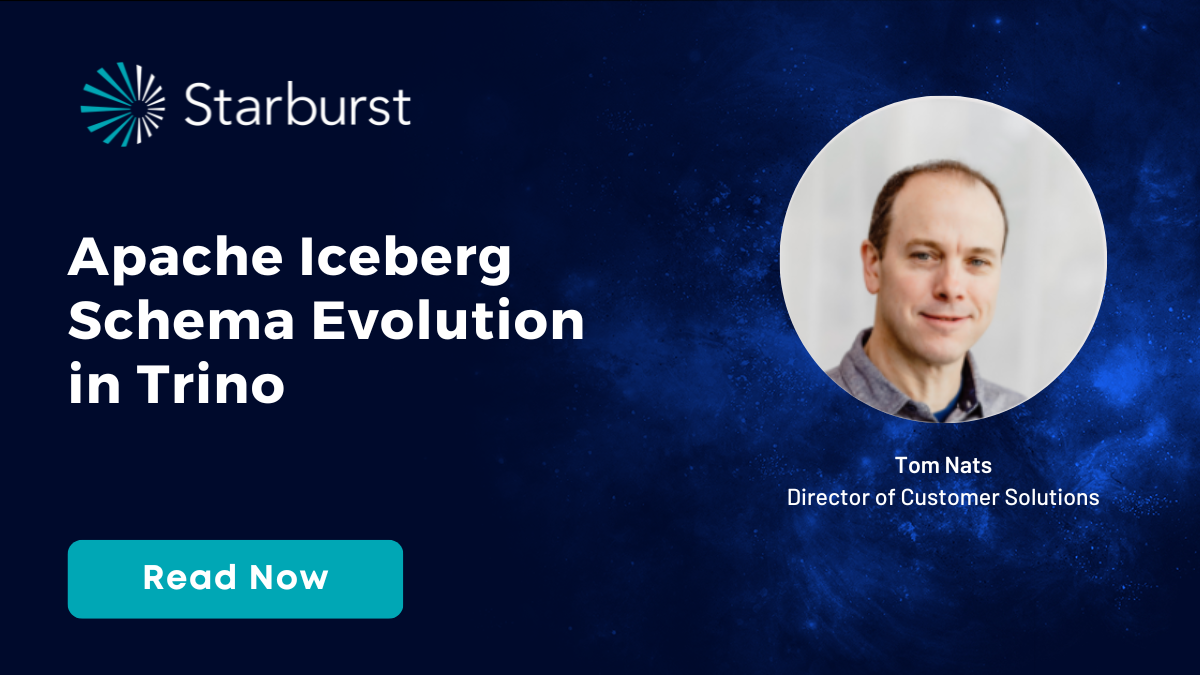
There’s also a dedicated tool to sync Hudi table schema into Hive Metastore.
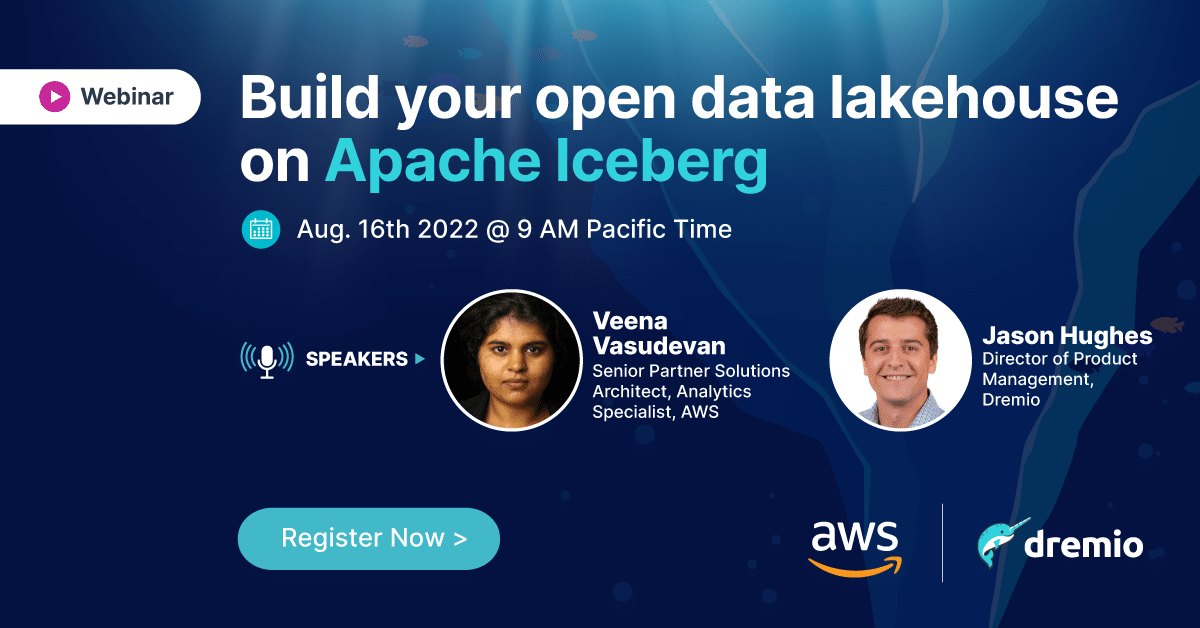
Reading data from Apache Hive, Apache Impala, and PrestoDB is supported. It also provides a Spark based utility to read from external sources such as Apache Kafka. It supports ingesting data from multiple sources, primarily Apache Spark and Apache Flink. Originally open-sourced by Uber, Hudi was designed to support incremental updates over columnar data formats. Finally, we’ll provide a recommendation on which format makes the most sense for your data lake.
#APACHE ICEBERG UPDATE#
Let’s take a closer look at the approaches of each format with respect to update performance, concurrency, and compatibility with other tools. Data & Metadata Scalability - Avoiding bottlenecks with object store APIs and related metadata when tables grow to the size of thousands of partitions and billions of files.Also handling potentially concurrent writes that conflict. Consistent Updates - Preventing reads from failing or returning incomplete results during writes.Atomic Transactions - Guaranteeing that update or append operations to the lake don’t fail midway and leave data in a corrupted state.

All three formats solve some of the most pressing issues with data lakes: It is inspiring that by simply changing the format data is stored in, we can unlock new functionality and improve the performance of the overall system.Īpache Hudi, Apache Iceberg, and Delta Lake are the current best-in-breed formats designed for data lakes. The outcome will have a direct effect on its performance, usability, and compatibility. When building a data lake, there is perhaps no more consequential decision than the format data will be stored in.
